Speed, scale, and collaboration are essential for AI teams — but limited structured data, compute resources, and centralized workflows often stand in the way.
Whether you’re a DataRobot customer or an AI practitioner looking for smarter ways to prepare and model large datasets, new tools like incremental learning, optical character recognition (OCR), and enhanced data preparation will eliminate roadblocks, helping you build more accurate models in less time.
Here’s what’s new in the DataRobot Workbench experience:
- Incremental learning: Efficiently model large data volumes with greater transparency and control.
- Optical character recognition (OCR): Instantly convert unstructured scanned PDFs into usable data for predictive and generative AI use cases.
- Easier collaboration: Work with your team in a unified space with shared access to data prep, generative AI development, and predictive modeling tools.
Model efficiently on large data volumes with incremental learning
Building models with large datasets often leads to surprise compute costs, inefficiencies, and runaway expenses. Incremental learning removes these barriers, allowing you to model on large data volumes with precision and control.
Instead of processing an entire dataset at once, incremental learning runs successive iterations on your training data, using only as much data as needed to achieve optimal accuracy.
Each iteration is visualized on a graph (see Figure 1), where you can track the number of rows processed and accuracy gained — all based on the metric you choose.
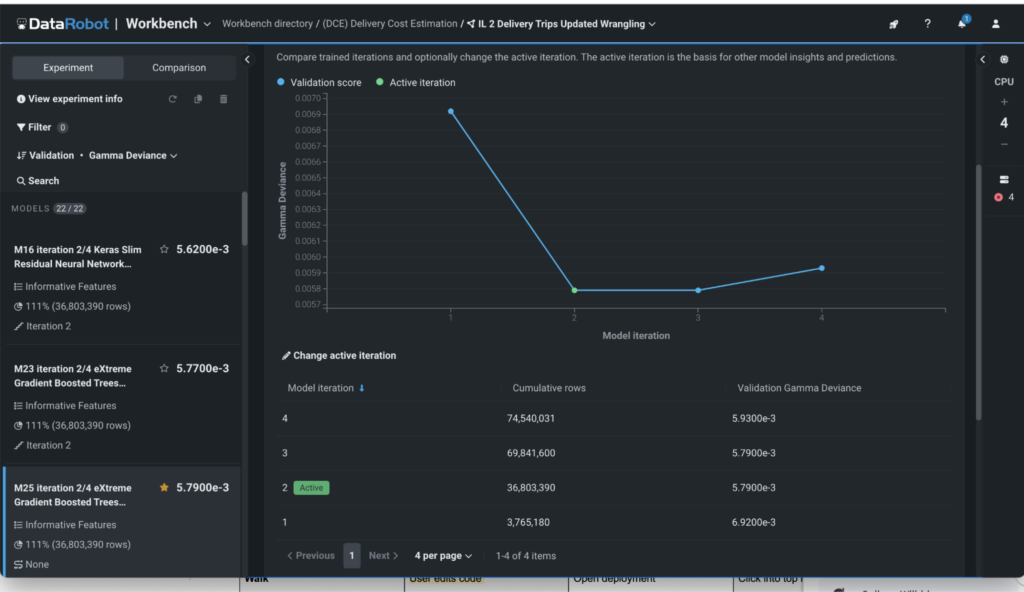
Key advantages of incremental learning:
- Only process the data that drives results.
Incremental learning stops jobs automatically when diminishing returns are detected, ensuring you use just enough data to achieve optimal accuracy. In DataRobot, each iteration is tracked, so you’ll clearly see how much data yields the strongest results. You are always in control and can customize and run additional iterations to get it just right.
- Train on just the right amount of data
Incremental learning prevents overfitting by iterating on smaller samples, so your model learns patterns — not just the training data.
- Automate complex workflows:
Ensure this data provisioning is fast and error free. Advanced code-first users can go one step further and streamline retraining by using saved weights to process only new data. This avoids the need to rerun the entire dataset from scratch, reducing errors from manual setup.
When to best leverage incremental learning
There are two key scenarios where incremental learning drives efficiency and control:
- One-time modeling jobs
You can customize early stopping on large datasets to avoid unnecessary processing, prevent overfitting, and ensure data transparency.
- Dynamic, regularly updated models
For models that react to new information, advanced code-first users can build pipelines that add new data to training sets without a complete rerun.
Unlike other AI platforms, incremental learning gives you control over large data jobs, making them faster, more efficient, and less costly.
How optical character recognition (OCR) prepares unstructured data for AI
Having access to large quantities of usable data can be a barrier to building accurate predictive models and powering retrieval-augmented generation (RAG) chatbots. This is especially true because 80-90% company data is unstructured data, which can be challenging to process. OCR removes that barrier by turning scanned PDFs into a usable, searchable format for predictive and generative AI.
How it works
OCR is a code-first capability within DataRobot. By calling the API, you can transform a ZIP file of scanned PDFs into a dataset of text-embedded PDFs. The extracted text is embedded directly into the PDF document, ready to be accessed by document AI features.
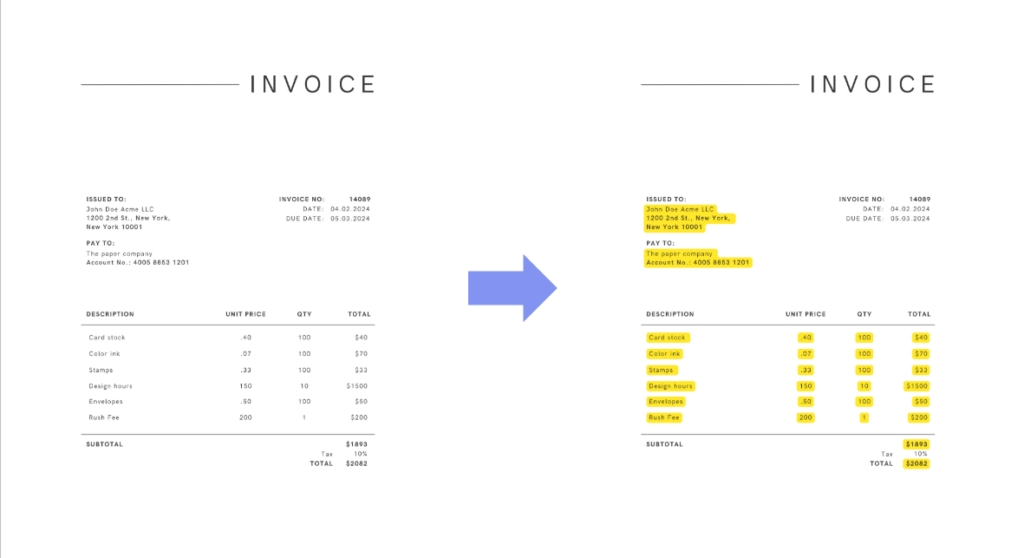
How OCR can power multimodal AI
Our new OCR functionality isn’t just for generative AI or vector databases. It also simplifies the preparation of AI-ready data for multimodal predictive models, enabling richer insights from diverse data sources.
Multimodal predictive AI data prep
Rapidly turn scanned documents into a dataset of PDFs with embedded text. This allows you to extract key information and build features of your predictive models using document AI capabilities.
For example, say you want to predict operating expenses but only have access to scanned invoices. By combining OCR, document text extraction, and an integration with Apache Airflow, you can turn these invoices into a powerful data source for your model.
Powering RAG LLMs with vector databases
Large vector databases support more accurate retrieval-augmented generation (RAG) for LLMs, especially when supported by larger, richer datasets. OCR plays a key role by turning scanned PDFs into text-embedded PDFs, making that text usable as vectors to power more precise LLM responses.
Practical use case
Imagine building a RAG chatbot that answers complex employee questions. Employee benefits documents are often dense and difficult to search. By using OCR to prepare these documents for generative AI, you can enrich an LLM, enabling employees to get fast, accurate answers in a self-service format.
WorkBench migrations that boost collaboration
Collaboration can be one of the biggest blockers to fast AI delivery, especially when teams are forced to work across multiple tools and data sources. DataRobot’s NextGen WorkBench solves this by unifying key predictive and generative modeling workflows in one shared environment.
This migration means that you can build both predictive and generative models using both graphical user interface (GUI) and code based notebooks and codespaces — all in a single workspace. It also brings powerful data preparation capabilities into the same environment, so teams can collaborate on end-to-end AI workflows without switching tools.
Accelerate data preparation where you develop models
Data preparation often takes up to 80% of a data scientist’s time. The NextGen WorkBench streamlines this process with:
- Data quality detection and automated data healing: Identify and resolve issues like missing values, outliers, and format errors automatically.
- Automated feature detection and reduction: Automatically identify key features and remove low-impact ones, reducing the need for manual feature engineering.
- Out-of-the-box visualizations of data analysis: Instantly generate interactive visualizations to explore datasets and spot trends.
Improve data quality and visualize issues instantly
Data quality issues like missing values, outliers, and format errors can slow down AI development. The NextGen WorkBench addresses this with automated scans and visual insights that save time and reduce manual effort.
Now, when you upload a dataset, automatic scans check for key data quality issues, including:
- Outliers
- Multicategorical format errors
- Inliers
- Excess zeros
- Disguised missing values
- Target leakage
- Missing images (in image datasets only)
- PII
These data quality checks are paired with out-of-the-box EDA (exploratory data analysis) visualizations. New datasets are automatically visualized in interactive graphs, giving you instant visibility into data trends and potential issues, without having to build charts yourself. Figure 3 below demonstrates how quality issues are highlighted directly within the graph.
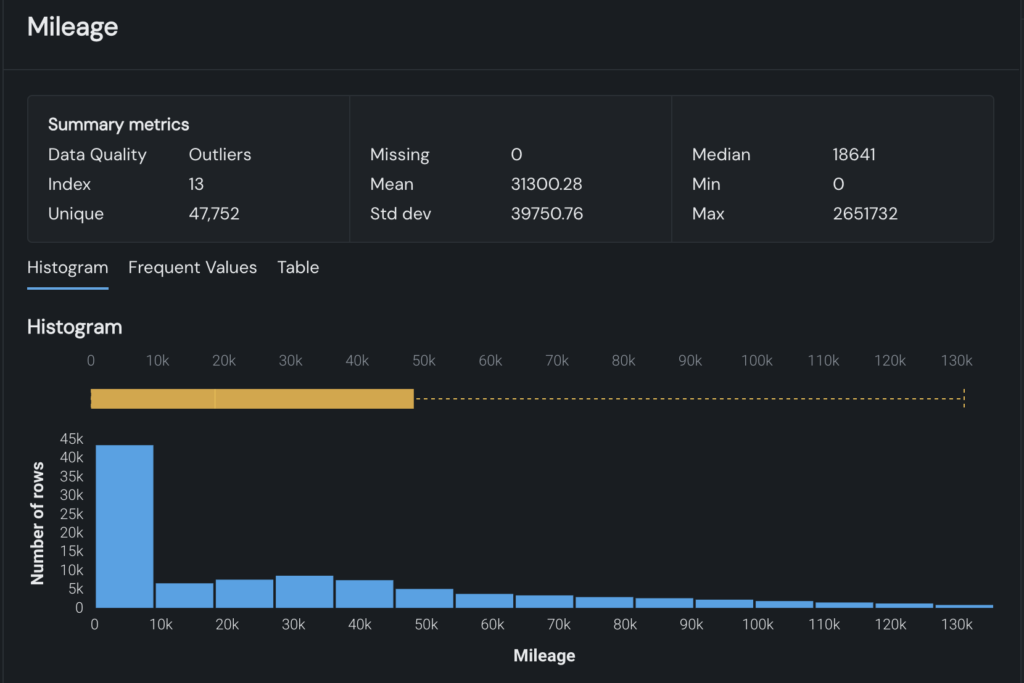
Automate feature detection and reduce complexity
Automated feature detection helps you simplify feature engineering, making it easier to join secondary datasets, detect key features, and remove low-impact ones.
This capability scans all your secondary datasets to find similarities — like customer IDs (see Figure 4) — and enables you to automatically join them into a training dataset. It also identifies and removes low-impact features, reducing unnecessary complexity.
You maintain full control, with the ability to review and customize which features are included or excluded.
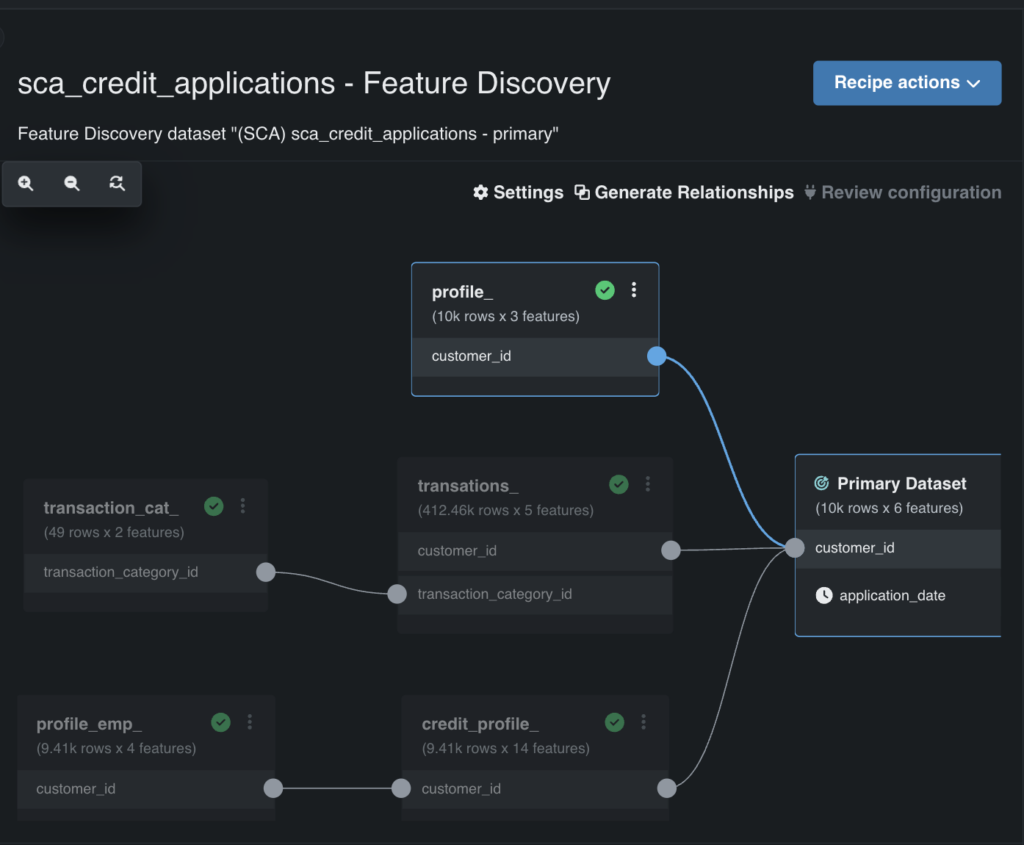
Don’t let slow workflows slow you down
Data prep doesn’t have to take 80% of your time. Disconnected tools don’t have to slow your progress. And unstructured data doesn’t have to be out of reach.
With NextGen WorkBench, you have the tools to move faster, simplify workflows, and build with less manual effort. These features are already available to you — it’s just a matter of putting them to work.
If you’re ready to see what’s possible, explore the NextGen experience in a free trial.
The post Accelerate data preparation and AI collaboration at scale appeared first on DataRobot.